Title: Federated Learning: From Algorithm and Networking Perspectives
报告题目:从算法和网络分析联邦学习的训练效果
Abstract:
Federated Learning (FL) has been considered as a more important decentralized machine learning framework, which can reduce the communication latency and protect the user privacy. However, FL has also some challenges to degrade the learning performance such as heterogeneous datasets and networking systems. In this presentation, I will present three of my papers to discuss how to solve these problems. For heterogeneous datasets, we leverage the state-of-the-art algorithm sharpness aware minimization to generalize the global model to protect the poor performance clients. For heterogeneous networking systems, we first proposed a new online client selection policy and then we developed a new multi-server FL framework.
联邦学习被认为是一种重要的分布式学习结构,它可以很好的降低通信延迟和保护用户的数据隐私。然而,联邦学习仍然存在一些亟待解决的问题,比如数据和网络的不平衡问题。在这次报告当中,我将针对于这两种问题利用我已经发表的论文给出解决方法。针对于数据不平衡问题,我们利用了一个新的Sharpness Aware Minimization 作为本地训练方法,使得全局模型更具有普适性并且很大程度的保护了那些数据极其偏离的客户端。
Bio:
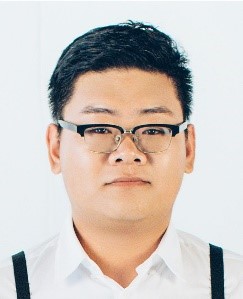
Mr. Zhe Qu is a Ph.D. candidate at Department of Electrical Engineering, University of South Florida. He got his B.S. degree from Xiamen University, and M.S. from University of Delaware in 2015 and 2017. His primary research interests are federated/decentralized learning, mobile network, and networking security. He has co-authored 12 publications in refereed journals and conferences, including ICML, ACM CCS, IEEE INFOCOM, NSDI, TMC, TDSC, TIFS and TPDS. In addition, he is the PC member and reviewer, including ICML, AAAI, INFOCOM, TIFS and TMC.
报告人简介:
曲哲,现就读于美国南佛罗里达大学电子工程系。他在2010年和2015年分别于厦门大学和美国特拉华大学取得本科和硕士学位。他的主要研究方向为联邦/分布式学习,无线网络建模和网络安全。他在ICML, ACM CCS, IEEE INFOCOM, NSDI, TMC, TDSC, TIFS and TPDS等重要国际会议和期刊上发表文章共12篇。同时,他也是多个国际会议的程序委员会委员和审稿人,如ICML, AAAI, INFOCOM, TIFS, TMC等。
报告时间:2022年9月22日 (星期四)上午8:30
报告地点:腾讯会议:322-134-599